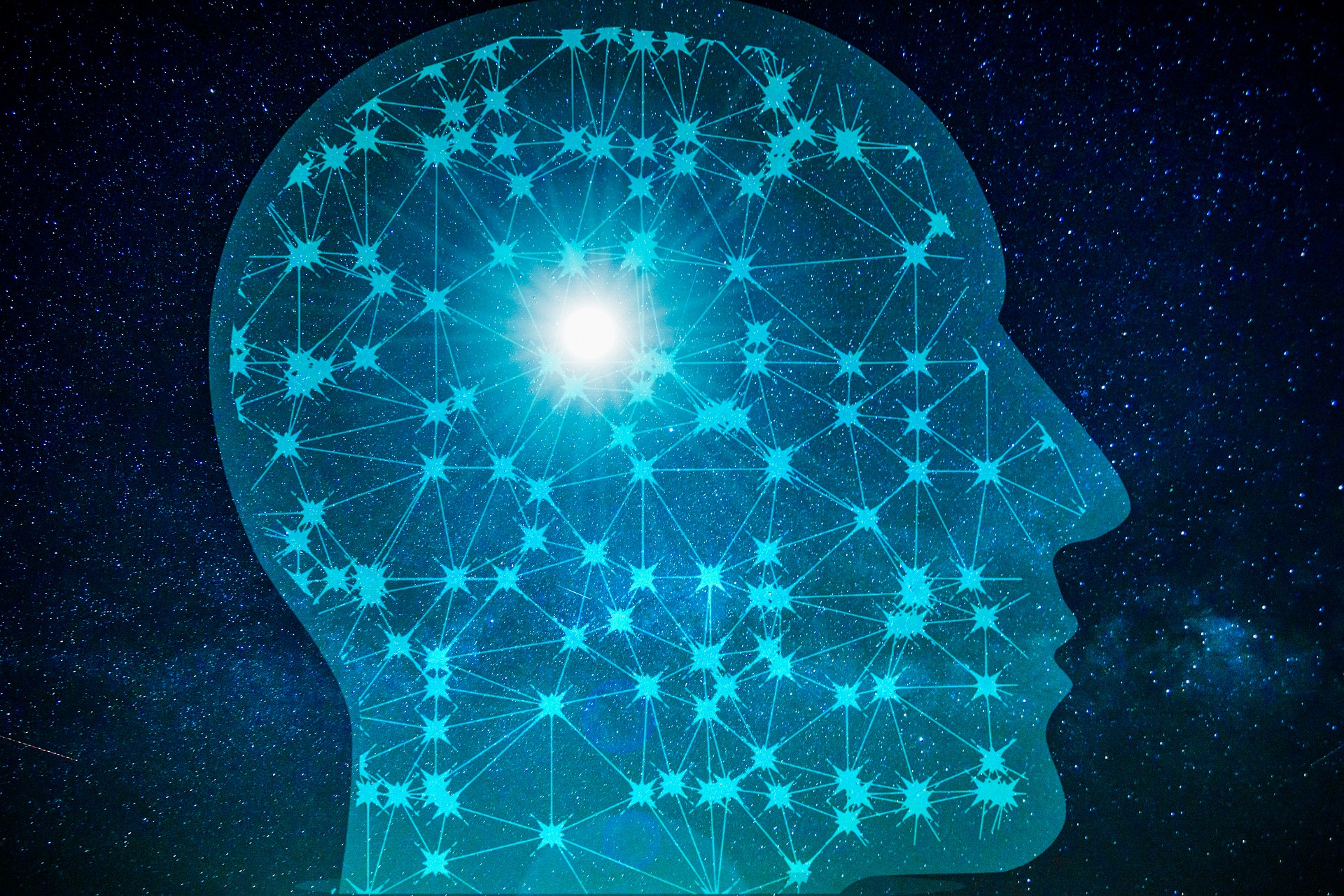
What Is Machine Learning?
By peterweddle
July 05, 2021
By Tim Dineen on the Recruitics Blog
Between artificial intelligence (AI) and machine learning, companies today are continuing to adopt and implement these business intelligent tools, as technology rapidly evolves, seemingly daily. 54 percent of business executives say that adopting AI in the workplace has led to a boost in productivity. AI and machine learning implementation seem to be ubiquitous, with 86 percent of CEOs claiming that AI technology is now mainstream in their offices and operations.
But, what is the difference between AI and machine learning? Since these tools and concepts often overlap, it’s easy to get confused. AI means “getting a computer to mimic human behavior in some way,” according to Oracle. On the other hand, machine learning is a subset of AI, consisting “of the techniques that enable computers to figure things out from the data and deliver AI applications.” In other words, machine learning “is the science of getting computers to learn and act like humans do, and improve their learning over time in autonomous fashion, by feeding them data and information in the form of observations and real-world interactions.”
Although much attention has circled around both AI and machine learning in the last several years, the technology has been around since the mid-1950s, with basic applications “like playing checkers and solving logic problems.” Machine learning, however, started to become more widely used in the 1980s, with a focus on “not just mimicking human behavior (AI) but mimicking how humans learn.”
Let’s delve deeper into machine learning and its applications.
WHAT IS MACHINE LEARNING?
Machine learning is “an application of artificial intelligence (AI) that provides systems the ability to automatically learn and improve from experience without being explicitly programmed. Machine learning focuses on the development of computer programs that can access data and use it to learn for themselves.”
Delving deeper into this AI application, machine learning uses a process beginning with data observations, allowing it to spot patterns. However, the primary purpose of machine learning is to “allow the computers to learn automatically without human intervention or assistance and adjust actions accordingly.” With machine learning, the AI application can access data, and then from it, learn for themselves.
Essentially, machine learning is really about the collection of data, first and foremost. And then using that data, and predictive modeling, to learn over time, spotting and understanding trends, allowing companies to make proactive uses of data. With its algorithmic-based decision-making, the entire genre of machine learning means that you're using historical data, or educated data of some kind, to inform and automate your decision-making efforts on behalf of your customers and clients.
Many different types of machine learning algorithms exist, with hundreds published each day. Typically, these algorithms are then categorized into learning styles, such as supervised learning, unsupervised learning, semi-supervised learning, or similar forms and functions, such as classification, regression, decision tree, clustering, or deep learning.
Regardless of learning style or function, all combinations of machine learning algorithms consist of the following:
• Representation (i.e., a set of classifiers or the language that a computer understands)
• Evaluation (i.e., objective/scoring function)
• Optimization (i.e., search method)
Based on the above learning styles and functions, business leaders are looking at real-time patterns with machine learning, indicating how things are changing in their field or the marketplace. For example, suppose a company wants to determine how to improve performance. In that case, it can use machine learning to determine—in real-time—that they aren’t getting the same amount of clicks, potentially resulting in increased pricing and expense.
From this data, companies can optimize their operations, allowing them to take advantage of trends that humans simply cannot spot. In addition, with predictive tools such as machine learning, leaders can gain focused and minute insight into the marketplace and industry, allowing them to make appropriate proactive shifts and adjustments boosted by machine power.
WHAT ARE SOME MACHINE LEARNING MISCONCEPTIONS?
Like other technological advances, many misconceptions revolve around machine learning. One common misunderstanding is that AI and machine learning are interchangeable, which we know from our discussion above, they are not. Further, another misconception is that machine learning cannot be trusted. This typically falls into the dangerous response of “we’ve always done it this way, so why change now?” Adopting and implementing new technology is critical for companies to “keep pace with dynamic environments.”
Another common falsehood is that with machine learning, you don’t need human intervention. Machines need training just as much, if not more, than human team members. They don’t know how to think without training, and certainly can’t improvise either. Machine learning does best at spotting patterns rather than “thinking” on its own. However, it would be best if you still had a balance between human involvement and problem-solving with the output of machine learning. AI and machine learning are not a “magic bullet;” instead, it’s a critical tool for running your business if used the right way.
Finally, the power of machine learning depends on the quality of your data. Remember the old business adage: garbage in, garbage out. The same applies to AI and machine data processes.
The misconception that machine learning is objective is patently untrue. Machine learning is not objective. It learns from “whatever biases you and your data have.” The better your data, the better your machine learning output.
Now, let’s learn more about how to use and implement machine learning.
HOW DO WE USE MACHINE LEARNING?
Big data and analytics indeed give us insights that we might not have otherwise had access to as humans. However, without making that data actionable, it isn’t easy to know how to use it.
With machine learning, companies can use years’ worth of historical data across a broad spectrum of clients, allowing them to take advantage of historical data and more recently available data to inform what the competition is like, for example, in a particular location or job type.
As another example, companies can instruct machine learning technology to store all data collected and analyzed where they can then make use of it, whether it's on a specific client basis or an aggregate client basis. Specifically, a company can track clicks and recruiting costs, comparing it to whether those interactions turned into job views, subsequent job views on different jobs, or applications, eventually leading to quality applicants or hires.
Machine learning is also intended to surface data so that employers can identify essential structures and patterns that may otherwise be obscured by outliers or challenges. These exceptions to the rule are obstacles for an algorithm and will decrease its prediction capabilities. While there are methods to ‘deal with’ the outliers, many employers rely on human insight and analysis to examine the data outliers further, thus making best possible outcomes possible for automated marketing platforms. The idea being that first you must remove those outliers, those ‘noises of nature’, in order to fit a model on the cleaned data.
For example, from the insights identified by machine learning, it might become apparent that competition increases seen in the data are a result of an intricate relationship between variables that humans aren’t yet aware of, or even able to see. Looking at the source level of these increases, companies can then determine what might impede their ability to budget appropriately or attract the clicks and attention they were looking for.
Since supply and demand change constantly, a machine learning system set up to look at a long period of historical data could ultimately get it wrong because of the continually moving marketplace—especially in a post-COVID world. Let’s look further at how best to use machine learning with supply-demand as well as competition.
HOW MACHINE LEARNING HELPS WITH THE SUPPLY-DEMAND AND THE COMPETITION
There’s no doubt that post-COVID, we’ll continue to see changes in supply demands, from supply chain management to hiring needs. For example, according to McKinsey, because of the impact of the global pandemic on low-wage workers, more than half of these workers “may need to shift to occupations in higher wage brackets [requiring] different skills to remain employed.”
Further, according to Gartner, post-COVID, “32% of organizations are replacing full-time employees with contingent workers as a cost-saving measure.” With machine learning and an abundance of data—both historical and current—recruiting professionals can better spot and anticipate trends moving forward, allowing employers to stay competitive in this new normal.
Because we are currently living in a very fluid work environment, accelerated by the pandemic, many companies are willing to spend varying amounts of money to acquire people in different markets based on their skillsets as well as their ability to generate revenue. With machine learning, recruiting professionals can more quickly identify trends from having an abundance of data, both from the consumer side demand and managing that supply of service providers.
Not only can these recruitment professionals more quickly identify trends, but they can also know how to better react to them. So if, for example, they are seeing more people churn in terms of the delivery supply side, if they start to see trends on the consumer demand side, or if they see spikes and patterns that mimic something seen in the past, they can be more proactive in sourcing talent.
For instance, if recruiting professionals can understand an organization’s shifting business goals and have access to both historical and current data, they can predict the inventory of job seekers in the marketplace. Further, from the trends spotted by the machine learning tools, recruiting professionals gain insight into better attracting top talent for a particular company’s needs, whether it is to bid more or be more aggressive in filling hiring needs.
THE IMPORTANCE OF MACHINE LEARNING AND WHY IT'S BENEFICIAL
According to Oracle, in recruiting, machine learning is already benefiting companies and recruiting professionals in three ways. First, machine learning tools can scan and screen resumes and applications, searching for specific keywords and “leveraging social data” to better identify job candidates. Next, AI chatbots can answer candidate questions, schedule interviews, and request additional documentation for the hiring process, freeing full-time employees to focus on higher-level tasks and reduce hiring costs. Finally, AI and machine learning can narrow “top candidates from a large pool using key attributes.”
Additionally, machine learning can better help companies source talent. According to Oracle, “[w]hile using machine learning can help reduce your recruiting cycle time, cost, and number of bad hires, you still need human intervention to manage the ‘candidate experience.’ Humans will still need to ensure the candidate experience is positive through actions such as frequent personal communication and high-quality and consistent interviewing techniques, regardless of the outcome for the candidate.”
Further, without machine learning, it would be difficult -- if not impossible -- to optimize talent sourcing across multiple platforms, multiple different types of job sites, dozens of various providers, and even different sourcing types, such as job boards, aggregators, social media, search engine, advertising, and so on. With machine learning, however, companies and recruiting professionals can drive the high-caliber candidate traffic efficiently and cost-effectively, potentially putting them ahead of the competitive curve.
By pairing robust technology with human experts in the industry, companies can benefit from the combination, making them efficient and highly competitive. One without the other would be trying to do something in a less informed way. Simply, if you don't have the data to back up your decisions, you're guessing. With new hires costing thousands of dollars, this is not an area in which to speculate.
WHAT ARE SOME COMMON MISTAKES WHEN USING MACHINE LEARNING?
When using machine learning, companies must look out for common mistakes and pitfalls. One primary trap involves the data itself.
Since machine learning models are predicated on data, it’s critical to examine your data regularly while looking for leakage. Reviewing your information regularly is essential to the success of your machine learning technology. Some companies check their performance data daily, looking for what’s going right and what should be addressed. For example, they’ll look at their data critically, asking, “How do we improve?” or “Can we be more efficient?” With machine learning spotting trends, leaders can look several steps down the road, anticipating market changes and proactive solutions.
Also, when implementing machine learning, you should hire professionals to help you model your technology, developing the appropriate processes along the way. Some strategies to develop thoroughly would be understanding the user, utilizing existing solutions, and performing regular failure analyses.
You also need to understand your goals for implementing machine learning in your company. For example, a company may quickly identify that an undersupply of talent is an issue when running data. However, leaders may not realize that an oversupply could also be problematic. For example, if you attract more candidates than available positions, you're going to anger people who also could be potential customers. Thus, oversupply may be bad for your brand.
Also, don’t just implement technology for the sake of implementing technology. You must understand its purpose, tailored to your goals and needs. However, don’t go the other route and decide you’re not going to implement machine learning at all. Doing so will require more employees and vendors, reducing any cost-effectiveness and potentially leaving you in the dark the next time the marketplace shifts. Remember, machine learning and human intervention are a balance one you must determine based on your particular needs.
MOVING FORWARD
New technological developments can often be intimidating. However, machine learning is not as scary as it sounds. Using data that’s available to you and then automating and scaling it, allowing you to make informed decision-making feasible, so why not?
If your company is looking for help implementing machine learning technology into your recruitment processes, contact Recruitics!